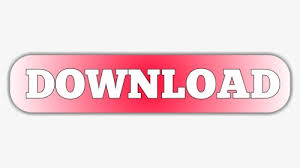
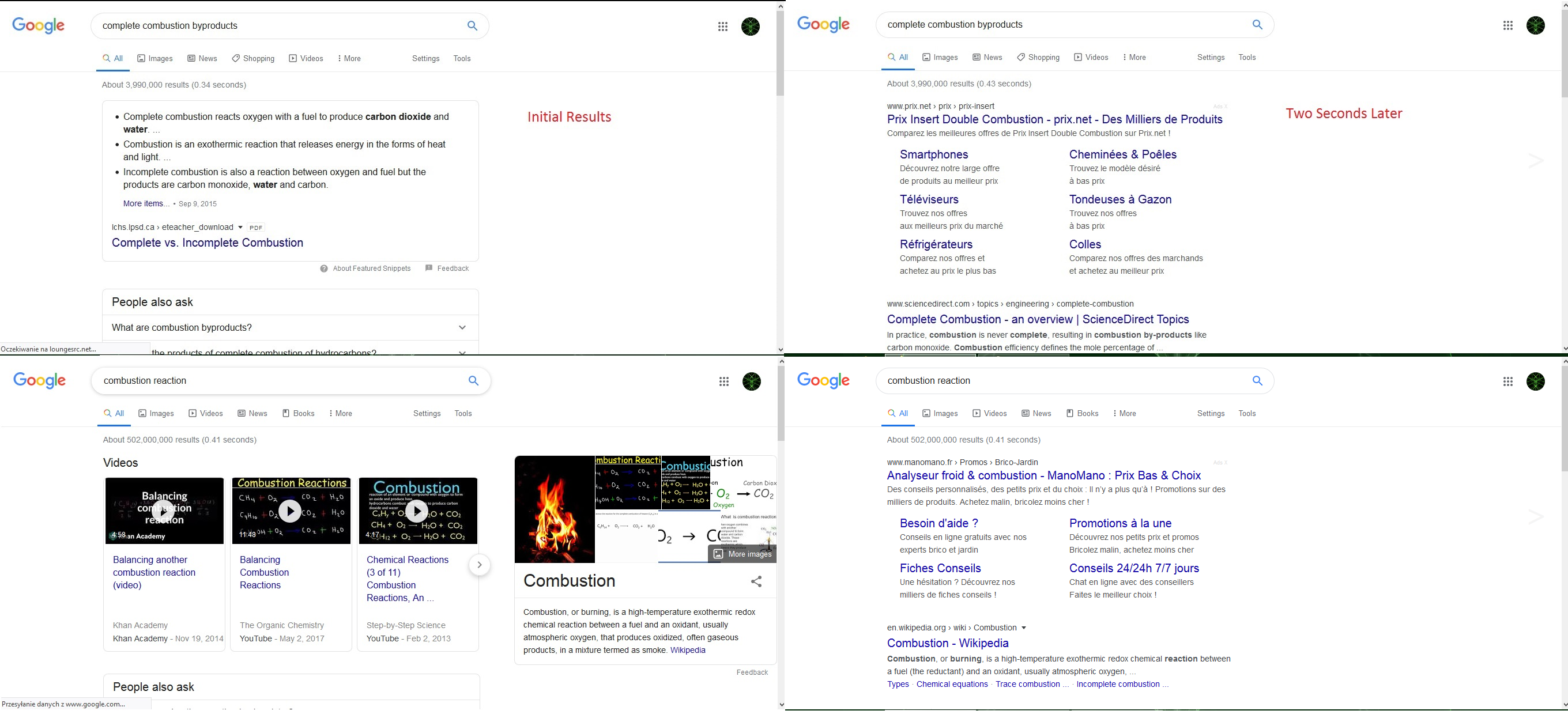
APT malware is one kind of advanced malware tailored for special targets, which has posed even more serious threats than the traditional malware. This malware is collectively called APT malware. However, unlike traditional network attacks, APT attacks will use some independent development malware to achieve specific purposes against different targets. Similar to traditional network attacks, APT attackers must use malware as attack weapons to attack in cyberspace. The purpose is to steal valuable confidential data or perform network espionage activities, which will cause severe harm thus, research on APT detection and prevention is urgent. They usually obtain huge financial or technical input, and they often perform long-term and complex attacks on certain targets. Recently, advanced persistent threat (APT) attacks have been continuously developed, and new types of APT emerge, posing severe threats and challenges to the network security environment in the present world. The experimental result shows that the proposed method can achieve 99.2% in distinguishing common malware from APT malware and assign APT malware to different APT families with an accuracy of 95.5%. Additionally, it can effectively intercept them in the initial invasion stage of APT to perform targeted defense against specific APT attacks by combining threat intelligence in public. This study aims to reduce the burden of network security staff from reviewing a large number of suspicious files when defending against APT attacks. Thus, we used transfer learning to perform multiple classifications of the APT family. By extracting the application programming interface (API) system calls, with the vector representation of features by combining dynamic LSTM and attention algorithm, we can obtain API at different APT families classification contributions trained dynamic. To defend against APT attacks and inquire about the similarity of different APT attacks, this study proposes an APT malware classification method based on a combination of multiple deep learning algorithms and transfer learning by collecting malware used in several famous APT groups in public. The model of similar studies also lacks an explanation about it. Unfortunately, the current research cannot effectively explain the relationship between the recognition, detection, and defense of APT.
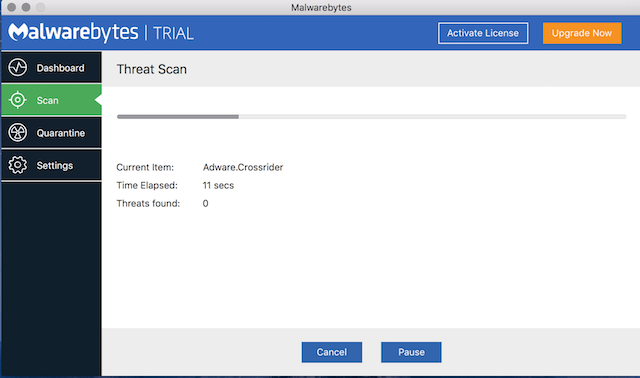
Therefore, we can enhance the understanding and cognition of APT attacks by comprehending the behavior of APT malware. Self-developed malware was usually used by advanced persistent threat (APT) attackers to launch APT attacks.
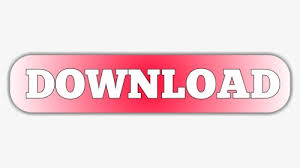